Navigating the AI Revolution in Smart Buildings: Opportunities, Roadblocks, and Strategies
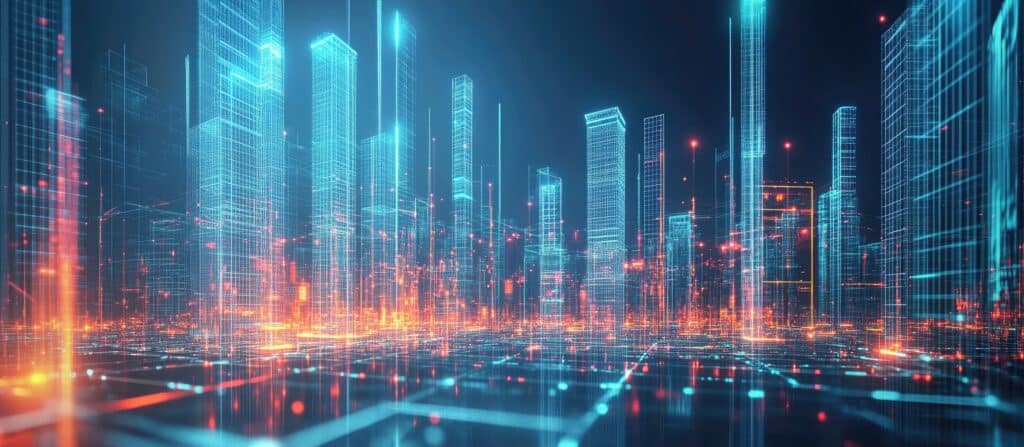
Authors: Amir Sekhavat (Superior Essex Communications), Dave Weatherly (Page), Jeff Ewing (5Q Cloud), Miguelangel Ochoa (ITTERA)
In modern buildings, automated smart building systems improve efficiency, enhance life and property safety, and elevate health and wellbeing for occupants. Building upon a decade of progress in integrating smart building technologies, the rise of Artificial Intelligence (AI) is now poised to redefine building “smartness.” Instead of relying solely on automated parameters and set points, building systems can leverage AI and machine learning to analyze vast amounts of building and occupancy data, enabling them to make intelligent decisions, self-adapt, and continuously optimize performance. While AI has the potential to bring building intelligence to a whole new level, it’s crucial to address the challenges and concerns that may hinder widespread adoption and take a strategic approach to implementation.
The Rise of AI in Smart Buildings
Conventional smart buildings integrate diverse management systems (lighting, HVAC, security) into a unified platform. IoT devices collect building data (status and performance) and interact with the integrated Building Management System (BMS), which regulates functional parameters within configured setpoints to ensure occupant comfort, satisfaction, and sustainable operation.
AI-based systems are an emerging subset of smart building solutions. While conventional smart building systems rely primarily on hardware and infrastructure (connected sensors and controllers), AI-based systems leverage advanced software algorithms and data analytics to optimize system responses. AI-powered smart building systems can apply traditional AI technology to analyze data, make predictions, and perform tasks or more advanced generative AI (GenAI) training models for informed insight and optimization.
Traditional AI follows predefined rules and thresholds, using structured data to recognize patterns, make predictions, and perform specific tasks. An example of traditional AI in a smart building would be an HVAC system dynamically adjusting to a pre-set temperature based on data from occupancy sensors. In contrast, GenAI uses sophisticated deep-learning algorithms to analyze massive datasets, learning patterns and granular insights to “generate” new intelligent outputs (predictions, solutions, and ideas) and create text, imagery, code, and audio. Large language models (LLMs) like ChatGPT, Google Gemini, and BingAI are types of GenAI tools that provide answers or perform tasks. An example of GenAI in a smart building would be analyzing vast amounts of occupancy, energy consumption, weather forecasts, and utility pricing to identify inefficiencies and generate corrective measures to reduce energy usage and cost. GenAI models improve with exposure to new data, allowing buildings to continually “learn” and adapt.
Opportunities with GenAI
GenAI technology opens up immense opportunities to optimize building operations, security, and occupant wellbeing far beyond what conventional smart building systems can deliver. Building owners, designers, and operators can monetize GenAI investments by reducing costs, enhancing tenant experiences, and generating new revenue streams. The following are just some ways that GenAI can drive profitability and long-term value for smart buildings.
- Power & Energy: GenAI-driven energy management systems significantly reduce utility costs by predicting and adjusting heating, cooling, and lighting needs based on energy usage, occupancy patterns, and environmental conditions. GenAI models can then use this information to create various simulations, assess the impact of different strategies and solutions, and make recommendations to optimize energy efficiency.
- Operations & Maintenance: GenAI-powered predictive maintenance analyzes historical and current operational conditions and learns patterns to identify failure conditions more accurately, simulate the impacts of failures, and generate maintenance schedules. This allows facility teams to resolve issues before they happen, increase the lifespan of assets, and minimize downtime and repair costs for building systems. GenAI can analyze occupancy, space utilization, and traffic patterns to recommend space usage and resource allocation, including identifying unused or underutilized spaces that can be eliminated, allocated for other needs, or leased for additional revenue.
- Health & Wellbeing: GenAI can monitor and analyze air quality data to optimize ventilation systems, identify potential issues, and improve overall air quality, contributing to occupant health and wellbeing. It can also leverage data from wearable devices and building sensors to provide personalized recommendations for occupant productivity, health, and wellbeing, such as suggesting optimal work environments, schedules, or exercise routines. Additionally, data collected through IoT sensors and GenAI analysis can be monetized by offering insights to third-party service providers or creating new revenue streams through tailored building amenities or subscription-based features.
- Safety & Security: GenAI-enhanced security solutions, such as intelligent surveillance, access control, and crowd control systems, can analyze vast amounts of biometric information, video footage, behaviors, language, traffic, and crime data to identify potential threats and vulnerabilities, simulate scenarios to assess risk, recommend resource allocation, and develop effective emergency response plans. These systems allow building owners to attract premium tenants seeking advanced safety features.
- Cybersecurity: While cybercriminals leverage AI to find vulnerabilities and launch sophisticated attacks, GenAI can also analyze attack patterns, network traffic, and configurations in smart building systems to enhance people and property protection. GenAI models can leverage this information to identify potential threats and vulnerabilities, recommend network and system settings, create response strategies, and manage and authenticate users.
- Digital Twins: GenAI technology combined with digital twins brings simulation and virtual representations to a new level. GenAI can accelerate the development of a digital twin and organize and feed live data and queries to the digital twin to develop more accurate models and simulation scenarios. Rather than just providing a simulation or replicating a system, GenAI allows digital twins to generate entirely new scenarios and make recommendations, such as for emergency response and evacuation in the event of a fire or cyber-attack vector simulations for tabletop exercises. Traditional AI is already proving valuable for facilities personnel training and assisted operations using augmented and virtual reality (AR/VR).
Challenges and Considerations
While GenAI technology offers significant opportunities for enhancing building intelligence, several challenges must be considered and addressed, including impacts on infrastructure, the need to ensure data security, a lack of skilled AI-based expertise, and employee concerns.
Impact on Communications Infrastructure
For GenAI to function, it needs a lot of data from smart building systems, devices, and sensors. Not only does this require more connectivity throughout a smart building, but transferring that data imposes significant demands on enterprise networks, particularly for speed and bandwidth. GenAI may accelerate the shift from copper to optical fiber networks that provide greater capacity and range. While most AI-powered systems will operate in cloud data centers that can manage the power and cooling required for advanced GenAI processors, some enterprises may also deploy GenAI in onsite edge data centers to enhance hardware security, protect proprietary data, and develop their own specific use cases. In this scenario, the onus is on the enterprise to ensure an infrastructure that delivers proper bandwidth, latency, power, and cooling.
The Need for Data Security
The vast amount of data collected and analyzed by GenAI systems increases the attack surface for cybersecurity threats. AI in a smart building also involves converging various data sources and sharing information across different platforms, which can lead to security vulnerabilities if integration is not managed correctly. Recognizing these risks and implementing stringent security measures to protect sensitive smart building information is crucial.
AI should be treated with the same level of scrutiny and policy enforcement as any full-time employee within the organization via rigorous compliance checks, continuous monitoring, and regular updates to security protocols. Encryption, access controls, and regular audits are necessary components of a robust security framework to maintain the confidentiality and integrity of data. However, crafting effective security policies for AI-powered smart buildings requires a deep understanding of the AI technology and the specific needs of the building environment. Organizations may lack the internal expertise to develop these policies on their own. Collaborating with external experts and forming strategic partnerships can provide valuable insights and ensure that security measures are comprehensive and current.
Lack of an AI Skillset
The commercial real estate industry faces a significant challenge due to a growing skills gap in AI and related technologies. Lack of expertise in AI ethics, prompt engineering, MLOps (Machine Learning Operations), and predictive maintenance and operations can hinder the development and maintenance of robust AI-based smart building systems.
Without the proper skillset, owners and senior executives may struggle to make informed strategic decisions regarding AI investments and implementations, leading to missed opportunities, inefficient use of resources, and deployment delays. This may result in higher costs and hinder the ability to keep pace with tenant and occupant expectations, creating a competitive disadvantage. Property managers and building engineers may also struggle to integrate AI and maintain the efficiency and reliability of smart building systems, such as ensuring compliance with privacy regulations, implementing proper security policies, and effectively adapting to new AI technologies for opportunities like predictive maintenance and energy management.
Employee Concerns
The pressure to quickly acquire new skills can impact employee morale and retention. Employee ethical concerns such as the potential for AI to displace jobs, biased decision-making in AI algorithms, data privacy violations due to excessive data collection and improper handling, and lack of transparency about how AI will be used must also be addressed. Providing continuous learning opportunities and ongoing communication about AI systems and their oversight are essential to mitigate these concerns.
Strategic Implementation of AI
Although still in its initial stages, GenAI adoption will be increasingly essential for enhancing building intelligence. However, many organizations need clarity on strategically integrating AI technology into their smart building systems, effectively creating an AI policy framework, and ensuring they have access to the interdisciplinary skills in data science and systems integration needed to develop and maintain robust AI systems.
Developing an AI strategy requires a structured, methodical approach to ensure success and avoid financial risk, especially given potentially high startup and ongoing costs. Before moving forward with an AI strategy, it’s imperative to set clear goals and metrics for success in line with business objectives and budgets, demonstrate measurable value, and assess the impact of potential risks on total cost of ownership.
Once the decision is made to pursue AI, users can build their own AI models using fully customizable systems, purchase a pre-trained system, or take a hybrid approach. Building your own AI model allows for greater customization, control over data privacy, and the ability to tailor solutions to specific needs, but it also involves significant investment, technical expertise, and prolonged development timelines. Widely used by startups due to its affordability and accessibility, buying a pre-trained system reduces cost and speeds deployment but comes with potential data privacy concerns, inaccuracies, and exposure to copyright violations. A hybrid approach combines the benefits of build and buy models, starting with a pre-trained model and customizing it with proprietary data for unique use cases. The hybrid approach could be ideal for smart buildings looking to leverage AI technology as it allows businesses to retain control over their data while leveraging external expertise, making it suitable for task-specific applications.
Regardless of the type of AI system, a robust, configurable policy framework is essential before deploying AI in operations. As technology and AI evolve rapidly, this framework must be continuously monitored, updated, and refined. It should cover:
- Data Management: Processes for collection, organization, and updating
- Model Oversight: Regular performance tracking, bias mitigation, and addressing inaccurate responses (e.g., false or misleading information known as hallucinations)
- Legal Compliance: Adherence to data security and regulatory standards
- System Integration: Seamless incorporation into existing IT infrastructure
Last but not least, ensuring the required skillset is necessary. Organizations may need to appoint roles like a Chief AI Officer or AI Ethicist to set goals, assess risk, choose a system, develop a framework, and oversee development, operation, and maintenance. Whether a GenAI model is external or internal, data scientists and prompt engineers are essential to developing, operating, and maintaining systems. Data scientists are highly educated individuals (typically a PhD) who oversee data collection, modelling, formatting, storage, interpretation, and management, including removing erroneous or biased data and updating the AI model regularly. Prompt engineers are computer science experts who design informed, precise inputs to optimize the AI model’s outputs, guiding its responses to ensure relevance and accuracy.
To remain competitive and innovative, organizations need a multidisciplinary approach to ensuring the proper skill set, including investing in continuous targeted training programs to advance existing talent, fostering partnerships with solution providers, and hiring specialized talent when necessary.
Key Takeaways
GenAI is poised to revolutionize smart buildings, offering significant opportunities for optimization, cost reduction, enhanced wellbeing, and new revenue streams. Through technologies like digital twins, GenAI also allows buildings to continually learn and adapt, allowing owners and operators to stay ahead in the evolving landscape of smart buildings. However, challenges related to communications infrastructure, data security, skills gaps, and employee concerns must be addressed.
A strategic approach encompassing careful planning, robust policy frameworks, and workforce development is crucial for successfully integrating AI and realizing its full potential in smart buildings. This multidimensional approach ensures operational excellence and establishes a sustainable framework for future innovations, allowing the commercial real estate industry to securely embrace the GenAI revolution and fully capitalize on its transformative potential.
This blog was developed by members of the TIA Smart Building Program Workgroup. This workgroup includes participants from all aspects of the smart building ecosystem to bring objective, holistic, and technology-neutral criteria for the industry to leverage in verifying the performance of smart buildings.
To participate in TIA’s Smart Building Program, contact membership@tiaonline.org
The ideas and views expressed in this guest blog article are those of the authors’ and not necessarily those of TIA or its members companies.